Category: Digital Transformation
How to Attain More Business Value with AI Implementation
The potential of Artificial Intelligence (AI) is being harnessed by businesses to implement automation across several industries and to augment their efforts to provide stellar customer experiences. The incomparable precision, speed, and accuracy of AI-enabled solutions are driving an AI revolution and this blog will discuss five specific ways you can attain business value with AI adoption.
AI: A new approach to customer experience
IBM, one of the leading universal AI champions reveals that 74% of customer experience executives feel that AI will change how customers view their brand. AI provides customers with an experience that appears very similar to human-like interaction. AI-powered apps are highly efficient in enhancing customer interactions.
Automation of “the customer experience journey” through AI enables your business to personalize your marketing campaigns, which will boost the email opening rate. Among other things, AI provides improved customer insights and better customer satisfaction which results in increased conversion and strengthens brand loyalty.
Related Reading: How AI is Redefining the Future of Customer Service
Steps to Gain Business Value with AI Adoption
A study from Market Research Engine estimates that the Artificial Intelligence Market is expected to exceed more than US$ 191 Billion by 2024. The vast growth of information in huge quantities, growth in the adoption of cloud-based applications and services, and an increase in the demand for intelligent virtual/ personal assistants are the major driving factors behind the booming AI market.
Unfortunately, many are still unsure of how to adopt AI, and how it can be used to yield maximum results. We present you these 5 steps to adopt AI which will help you gain the maximum business advantage.
1. Map out a clear customer-centric strategy
The best way to do this is to analyze recent customer journeys with your brands such as discovery, presales, sales, and customer service. Such analysis will help you understand the experience your customers are having with your brand. Research director at Gartner, Olive Huang said, “Your business results depend on your brand’s ability to retain and add customers.” Hence it’s important to deliver a highly personalized experience to every one of your customers. Once that strategy is crafted, it can be delivered through AI.
Related Reading: 6 Ways Artificial Intelligence Is Driving Decision Making
2. Diagnose the problem
Just as the prudent diagnosis of a disease is crucial to helping a patient improve their health, finding out your specific business problems will help you use AI to improve business value. Organizations need to be clear about what AI can help them accomplish. Once the problem is identified, aligning AI to business priorities becomes easier. The beauty of AI algorithms is that once algorithms are enabled to solve one aspect of the business successfully, it can easily be adapted for use in other aspects of the business too.
3. Establish a governance program for customer experience
Before you begin your journey with customer experience, make sure to establish a governance program. The benefit of having a central team working on the AI project is that you can integrate program oversight with a complete comprehension of AI-related initiatives. This is true even when your customer experience teams are just in the learning process. Build a program to supervise development activities and business implications and set brand guidelines for AI technologies such as NLP. Also, establish standards to gauge the impact of customer experience initiatives and its correlation with ROI.
4. Bring the best onboard
Creating a custom AI solution is recommended over buying it readymade. It is important however that you bring in the best talent. Bringing in a team of dynamic people who can create exciting ways to engage with the customers sends a powerful message to your competitors. It will also put your organization on the radar as a tech-savvy innovator.
Read our latest white paper: How Could Your Business Use AI to Achieve Higher Profits & Growth
5. Prepare to store
A business that fails to look into the future fails to grow. Gathering relevant data allows organizations to derive greater benefits far into the future. Meaningful data help AI systems in achieving your organization’s objectives. Insufficient data could compromise the accuracy of AI applications, so get your team to build relevant data including cases and codes.
Drive Business Value With AI
Organizations are adopting AI faster than anticipated. To gain long-term and sustainable business value from Artificial Intelligence, you need to develop a robust implementation approach that takes into its fold, even the minute aspects of your enterprise. Contact us to adopt the power of AI into your business.
Stay up to date on what's new
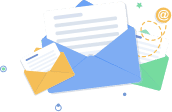
Featured Blogs
Stay up to date on
what's new
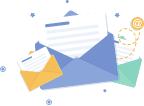
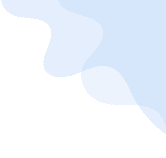
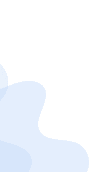
Talk To Our Experts
How to Determine If Your Business Process Qualifies for Automation
A large number of businesses are adopting Robotic Process Automation (RPA) today to drive their critical enterprise operations quickly and affordably. While RPA offers a wide array of automation capabilities, you need to first identify which of your business processes are ideal to be automated so that your business enhances operational efficiencies to deliver positive outcomes. For instance, some companies achieve better outcomes when implementing rule-based automation when compared to non-standardized, variable types. Forrester recently reported that RPA Market will reach USD 2.9 billion by 2021, which makes it one of the most sought-after enterprise technologies. The picture is clear: it is crucial for companies to know the different ways to determine which of their business processes need automation.
This post attempts to walk you through the major questions and answers which can help you decide the candidature of a process for Robotic Process Automation:
Criteria to determine if a process is fit for RPA automation
Deciding whether a business process requires RPA implementation or not depends on two criteria:
- Process Fitness
- Automation Complexity
Process Fitness
To determine if a process is reasonable enough to offer tangible results by implementing RPA, it is first critical to understand the process type being considered. RPA can automate only clear and well-defined processes. For this, it is first required to know which category the workforce’s tasks belong to:
Repetitive/ Automatable processes
Automatable processes relieve the human workforce from performing repetitive tasks such as clerical and data entry works or data manipulation tasks. Automating such redundant tasks allows human workers to focus on core, value-adding functions.
We can differentiate four types of processes:
- Manual and Non-Repetitive Processes: The steps are performed by humans and are performed every time the process is executed.
- Manual and Repetitive Processes: The steps are performed by the user. A few of these steps are the same every time they are executed.
- Semi-Automated and Repetitive Processes: A few of the steps in these processes are already automated, using Macros, Outlook rules, and so on.
- Automated Processes: These processes are those that have already been automated by technologies other than Robotic Process Automation.
While the above four are somewhat repetitive/ automatable, there is another category: Manual Or Non-Repetitive processes that are not great candidates for RPA. This is because these processes need to stay manual or are non-repetitive due to the high exception rate or factors that cannot be integrated into business logic.
Rule-Based Processes
For a process to be automated, it should be rules-based. Human-made rules are applied based on which the system executes the process. Rules can be related to storing, sorting, and manipulating data. The rules-based system is a logical program that uses a predefined logic to interpret data or make decisions. These processes are always easy to use and understand. The rules-based processes have an exception rate which is either low or can be included in the business logic as well.
Standard Input Processes
Standard input processes are those that need to be either electronic/ easily readable or are readable using a particular technology that can be associated with RPA. An example of a standard input process is the OCR. The Optical Character Recognition or OCR algorithms have processes whereby printed or handwritten documents are scanned and analyzed automatically and the text data is converted into editable formats for efficient processing. Using OCR is a much more reliable way to automate tasks such as invoice processing.
Stable Processes
Stable processes are processes that have remained the same over some time and no changes are expected in the coming months. These processes are good candidates for automation, provided they meet with other critical criteria as well. The output of these processes is fairly predictable.
Related Reading: Robotic Process Automation: Choosing The Right Solution For Your Business
Automation Complexity
It’s also crucial to analyze the complexity of a process to see if it’s fit for automation. The complexity of deciding to automate a process depends on several factors such as the number of applications or systems, the number of times human intervention is required, or even the number of steps required to execute the given task.
Following are some of the factors you need to look at:
- Number Of Screens: RPA in this scenario works by programming the robot to perform functions at the screen level. That is, when the screen changes, the logic is taught. The number of screens is directly proportional to the elements to be captured and configured. For instance, the higher the number of screens, the larger is the number of elements to be captured and configured before process automation.
- Types Of Applications: There are different types of applications. Some can be easily automated such as the Microsoft Office Suite or Java. Some other processes require complex automation effort, such as Mainframe applications.
- Business Logic Scenarios: The complexity in automating a process increases with the increase in the number of decision points within the business logic.
- Types And Number Of Inputs: Standard Inputs are desirable. For instance, an invoice that is a standard input and needs to be configured for each supplier will be impacted by automation. On the other hand, non-standard inputs will have varying complexity grades. Among these, free text is the most complex one.
Using the above-mentioned four factors, the processes can be split into four major categories:
-
No RPA Processes
These are processes in which change happens frequently. The system environment is volatile and multiple non-digital or manual actions are needed.
-
Semi-Automation Processes
Semi-automated processes are fragmented down into multiple steps that are automated. These steps include the ones that need to be manual such as the validations of physical security tokens.
-
High-Cost RPA Processes
These processes are digital and can be automated. High-cost RPA processes either make use of some complex technologies such as Optical Character Recognition or OCR or require advanced skills in programming.
-
Zero-Touch Automation Processes
These processes are digital and involve a highly static system and process environment. This makes it easy to be broken into instructions and define simple triggers.
The Stages In RPA Implementation
RPA offers a multitude of ways in which the degree of automation can be increased. There are commonly 6 stages involved in RPA implementation:
- RPA Preparation: Here, the processes are defined, assessed, prioritized, and then the plan is implemented.
- Designing The Solution: Here, each process to be automated is documented ( as “as is” and ”to be”). The architecture is now created and reviewed. This is followed by the preparation of test scenarios and environments. With this, the solution design is now created and documented for each process.
- Building the RPA: In RPA building, the processes are automated, the workflows are tested, and validated. This is followed by the preparation of the UAT.
- Testing the RPA: The UAT is now performed, followed by the debugging of the workflows. The process is now ready to be signed off.
- Stabilizing the RPA: In this phase, initially, the Go-Live is prepared. The process is then moved to production, monitored, and measured. The lessons learned are now documented.
- Constant Improvement Phase: This phase involves the assessment of process automation performance. This is followed by tracking the benefits and managing the changes.
Download our latest white paper: How RPA Simplifies Business Operations
Utilizing RPA Effectively
Every company planning to use/ already using RPA applies automation to fulfill different operational goals. While businesses at the beginning of their RPA journey want to uncomplicate and rapidly execute their workflows, the veterans in RPA might wish to expand the scope of their existing automation solution and improve their regulatory compliance. Each one wants to leverage technology differently.
Whatever your goal is, Fingent helps businesses in leveraging RPA to deliver high business value, drive significant cost benefits, and enable technology to have a positive impact on your operational activities. Get in touch with us to know how we can realize your automation goals.
Stay up to date on what's new
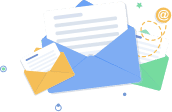
Featured Blogs
Stay up to date on
what's new
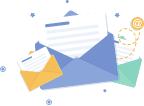
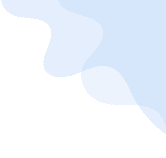
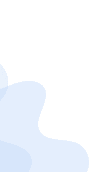
Talk To Our Experts
5 Tips To Select The Ideal Chatbot For Your Business
Chatbots have opened up a whole new realm of communication between humans and machines. They enhance a company’s customer service and improve operational efficiency, driving better engagement, reduced churn rates, and overall sales growth. They have become immensely popular and their popularity only continues to increase. It is clear that the use of chatbots is imperative for your business success.
In this blog, we will take a look at the types of chatbots available and how to wisely select the chatbot that suits your business.
The Wide Array of Bots
Studies predict that by 2021, more than half of the enterprises will increase their investments in chatbots, creation than traditional mobile app development. Customers would prefer to get real-time answers from bots on a company website.
Chatbots can do just about anything. They can help you deliver a surprise gift to someone you love. They can also help you break up with your lover and much more!
Broadly, chatbots can be classified as follows:
- Action Chatbot: In order to follow through with a specific action, this type of chatbot requests relevant data from the customer.
- Social Messaging Chatbot: It utilizes social messaging platforms and allows customers to interact with the chatbot directly on social media.
- Scripted Chatbots: It uses a predefined questionnaire to interact with the customer.
- Natural Language Processing (NLP) Chatbots: Being an application of AI, NLP enables chatbots to understand the written or spoken language and come up with the best response.
- Contextual Chatbots: It is the most brilliant of all chatbot types. Since it is based on artificial intelligence and machine learning, it can self-improve over time.
Tips To Choose A Perfect Chatbot For Your Business
As a communication agent, chatbots play a vital role in automating mundane tasks in an “always-on” work environment. Chatbots can handle day to day queries until an emotive or complex issue arises, which might require the intervention of a trained human agent to address it.
Related Reading: Capitalizing on AI Chatbots Will Redefine Your Business: Here’s How
Here are a few pointers to select the perfect chatbot for your business needs:
1. Think about your target audience
Like every business that has a target customer, the chatbot too must have a target audience. It is important to remember that the chatbot should serve as the bridge between you and your customers. The bot should be able to understand the preferences of your customers and cater to their convenience.
2. Define objectives
Identifying and narrowing the specific tasks or areas you want to automate would yield maximum benefits. There are a few points that could help your business define those objectives. Carefully consider factors such as the platform where the chatbot would be used, the queries it would answer, the queries it would direct to a human customer care executive, and how it would manage the hand-over process smoothly.
3. Define your value proposition
The value proposition involves ensuring that the most vital factor of your business, is given prime consideration. It determines whether your customers will come to you or go to your competitors. A higher value proposition might require AI or ML capabilities; so gauge and determine your value proposition to select the right chatbot that fits your budget and your business needs.
4. What is your response speed?
According to the 2018 State of Chatbots Report, customers want quick and easy answers. Customers might get frustrated if the answers are delayed. The appropriate selection of chatbots can help you avoid such kind of delays effectively. When dealing with a complex issue, ensure that your chatbot is capable of collating information quickly without delay. If there is a need to hand over the query to a human customer care agent, it should be done seamlessly and fast.
5. Evaluate features and functionalities
Evaluation aids your business in identifying the essential features and functionalities required from a chatbot to run your business successfully. To begin with, you could create a set of standards that would analyze all solutions. Decide on which features are required, such as NLP, integrations, contextual awareness, analytics, and so on. Proper documentation is required while evaluating the features. Such a candid evaluation helps a business choose the right chatbot that could be fine-tuned later or could self-learn.
Download our case study: Using chatbots to create an enhanced and engaging learning experience
Make Your Business Chatbot Ready
In the 24/7 era where customers want instant services, chatbots help businesses to keep pace with such expectations. By evaluating your own objectives and keeping in mind your customers’ expectations, your business can maximize the benefits of chatbot technology. However, choosing the right chatbot that fits your organizational needs and implementing it without any flaws require a good deal of expertise.
Our team at Fingent has been doing amazing things with Chatbots for our clients. Recently, we provided a matured chatbot assistant technology to a client, which provides comprehensive user intent identification and processing as well as satisfactory response according to the user query. Chat with us to identify the best chatbot solution for your business, and learn how we can implement it for you quickly.
Stay up to date on what's new
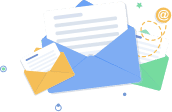
Featured Blogs
Stay up to date on
what's new
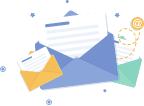
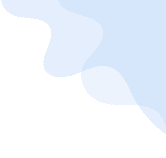
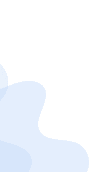
Talk To Our Experts
Overcoming Data Challenges in Insurance: How Blockchain Helps
We live at a time when the global data regulators are increasing their data security requirements across businesses and industry verticals. Data being the most powerful asset of insurance providers, insurance companies worldwide have started implementing steps to secure confidential and sensitive information with the help of advanced technologies like blockchain. The vast growing data holds an immense potential to transform insurance companies, but managing the data coming from multiple sources throws up a lot of challenges. Here’s how blockchain helps in overcoming the data challenges in the insurance industry.
Data Challenges in Insurance Vertical
As you know, insurance is a data-centric service. Let it be life and annuity, property and casualty, risk, loss, or claims, insurers are dependent on large volumes of data for various workflows and operations. With increased data flow comes high risks associated with data storage. A wide range of mobile-friendly options is now made available for consumers by the insurance providers to share their personal data. When insurers request access to customer-specific information, they must ensure the authenticity and security of the data collected. The rapid adoption of digital transactions has left insurers in a complex situation to identify new ways to streamline processes and secure sensitive information.
A recent report from McKinsey states that an estimated 5 to 10 percent of all claims are fraudulent, which costs the US-based non-health insurers more than USD 40 billion per year. Besides handling huge amounts of data, the insurance industry struggles with complex compliance issues, third-party payment transaction challenges, slow growth in matured markets, and fraudulent claims activity. Insurers can overcome these obstacles by gaining more accountability, transparency and superior security provided by the blockchain tech.
Related Reading: Top Five Barriers To Growth and Adoption Of Virtual Customers
How Blockchain Rescues Insurance Companies
For an insurance provider to capitalize on the large volumes of data generated, the data must be analyzed in depth to derive useful insights. This process first requires the data to be stored in a trusted manner. Blockchain allows an action to be performed using a predetermined set of rules that are based on threshold triggers in the data. This huge potential of blockchain plays a major role in saving cost and time as well as increasing your business value.
Blockchain makes use of advanced cryptographic techniques, also known as distributed ledgers, to store data. A secure ledger of data is created which cannot be modified, added, or removed without proper authorization. The advantage of Blockchain that is leveraged by the insurance industry over other technologies is the data security it offers along with clear audit trails. The data stored in a blockchain is immutable as it resides in a distributed ledger that executes transactions using a consensus-based mechanism. Each action is recorded permanently with a time and date stamp, such as titles or document histories, which improves the storage’s credibility.
Securing Insurance Data
Blockchain offers the following capabilities to handle data challenges in the insurance industry:
1. Distributed Network – Every participant in the blockchain network has a full copy of the ledger. This ensures transparency among all those who’re part of the insurance workflow.
2. Programmable Network – Blockchain is programmable with the use of smart contracts, also known as crypto contracts. These are programs that control the transfer of digital currencies and assets between parties directly. They also execute transactions and simplify repetitive processes with real-time auditing and assessment of risks.
3. Time-stamped Transactions – Transaction timestamps are recorded in a block which ensures no data is corrupted/ tampered.
4. Secure – Records are individually encrypted and cannot be manipulated.
5. Irreversible – Validated records are immutable, that is, they cannot be changed.
6. Anonymous/ Pseudonymous Identity – The identity of participants is either anonymous or pseudonymous, which ensures the confidentiality of customer data.
Related Reading: How AI and Machine Learning are Driving Cyber Security in FinTech?
The Upcoming Trends
Decentralized validation, redundancy (continuous replication of data), immutable storage, and encryption are the most important characteristics of blockchain that make it a viable option for insurance companies. Through decentralization, it enables faster and cost-effective peer-to-peer transactions, compared to the traditional methods.
Blockchain is expected to open new channels into different market segments and geographies. The Insurance industry is taking leaps and bounds to explore the many advantages of blockchain technology. An industry-wide collaboration among market players, technology evangelists, start-ups, and regulators can help create more potential use cases of blockchain in insurance, leading to operational transformations in a better regulatory environment. Just as the early bird catches the worm, getting involved in partnerships and industry changes at the earliest is key to shaping the future of the blockchain-insurance ecosystem.
Whether you need a detailed/crisp briefing on Blockchain and how the technology can add value to your insurance business, give us a call asap. Our expert will guide you on how to take advantage of blockchain in your business.
Stay up to date on what's new
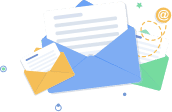
Featured Blogs
Stay up to date on
what's new
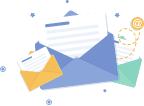
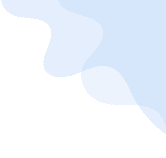
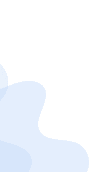
Talk To Our Experts
You are planning to build an app for your business. You zero in on the app features, then develop it and finally release your app to the respective stores. You actively monitor your app’s usage and analytics. Your users are happy and they actively use your app. After a while, you notice a dip in the curve.
Your app starts falling behind in-store listings, garners poor user reviews, becomes frequently prone to errors. Then one day you get an email from the respective app store that your app might be removed if it does not meet the latest requirements of the app store.
So, what went down?
Well, did you ever take the effort to continue maintaining the app? Delivering updates on a timely basis so that your app stays compatible with current requirements and is well ahead in terms of features, functionality, security, and performance is a vital part of app development.
You might have built the perfect app, but how long can it remain active and not pass out as obsolete without proper maintenance?
As an enterprise app development company, our experience has shown us that creating and deploying an app is sensible and profitable in the end only if you tie it up with a regular update cycle. We put equal stress on pairing a solid maintenance contract having timely release cycles into every mobile app that we built and always insist on our clients on the importance of having one.
Ongoing maintenance remains indispensable in app development
In 2016, Apple made a relentless push clear its App Store for all outdated apps, delisting 50,000 apps according to a report by Sensor Tower. It further revealed that 51 percent of apps haven’t received any updates in a year.
Source: Sensor Tower
Earlier, you build an app and launch it. You only need to focus on developing the app in the best possible way. However, things have changed. Ongoing maintenance is so important now that almost 80 percent of developers release some form of updates to their apps every month.
Performing maintenance constantly, reinvent your app’s functionality and fix any bugs or other latent issues. It’s unlike the build and deploy once approach that was employed traditionally.
To keep up with the ever-changing user and app store requirements, you should not only build, deploy and optimize your app for the store listing but also improvise it by regularly issuing new updates. Like a newly constructed house that requires additional investment and efforts at maintenance like cleaning, painting and other upgrades, your apps should similarly go through a regular upkeep cycle to keep it active.
Besides, setting up a maintenance plan is so important for your business as several external variables are prone to changes that can affect your app’s functioning. These include:
- Mobile hardware: As handset manufacturers keep releasing newer models each year, mobile apps can become incompatible with the latest mobile hardware, causing them to fail. With a maintenance plan, you can continuously deliver new updates that extend support to all the latest mobile hardware specifications.
- OS versions: Android and iOS are evolving with new version upgrades every year and your mobile app should take into account these changes to extend support and compatibility with upgraded OS versions.
- User Interface: Material Design, Google’s visual design language, marks the change from Skeuomorphic principles towards a fresh approach to user interface design. Keeping up with the current user’s preferences meant adopting newer UI/UX design standards for the app interfaces offering immersive digital experiences.
- Security: With data breaches now commonplace, you should integrate all recent security protocols into your apps to uphold privacy and security.
- Programming languages: Programming languages use to build apps also undergo changes and your apps should stay updated considering these variations.
- Software libraries: Third-party software libraries and dependencies used in apps go through frequent changes, which affects your app’s functioning and cause it to malfunction if they are not upgraded.
- Licensing: Apps that you build has to be tied down to licensing agreements and certifications. These agreements are a limited time offer and therefore need to be renewed periodically to keep the app intact and functional throughout its lifetime.
- Hosting infrastructure: The platform where your app’s database and backend are hosted such as AWS can also be subject to changes throughout the year.
Signing maintenance contracts save your app from getting outdated
Post completion, your vendor may ask about maintenance contracts for your app. Most businesses decline to sign up for a maintenance contract, because to them, the idea of paying extra amount for an already launched app sounds absurd and unconvincing. However, considering the necessity of app maintenance, it is mandatory that a maintenance contract is bought in and formulated alongside the actual development.
Once you sign up for a maintenance contract, you get the dual advantage of both support and regular updates. Every issue that crops up will be dealt with immediately by the development team once you enroll for a maintenance contract, thereby significantly reducing your app’s downtime. Besides, when platform changes occur, such as new OS versions or hardware, your app development partner can review them in advance and plan all future updates accordingly.
Understanding maintenance costs
Maintaining your app continually comes with its costs, which can vary depending on the scope and functionality of the app. Clutch, in one of their surveys, outlines that post-launch maintenance can cost anywhere between $5000 – $10,000, a year after launching the app, which varies based on the vendor opted.
However, maintenance costs follow the standard industry norm, which is 20 percent to that of the initial development cost. For instance, if your app costs about $10,000 to develop then maintenance would run around to $2000 a year. This is subject to vary as it takes into account several other factors like the type of app – native or hybrid, additional features – (push notifications, payment gateway), backend hosting platform, use of third-party analytics tools, etc.
So, what real benefits does your business gain by signing up for a maintenance contract with an app developer?
- Lower Uninstall Rates – AppsFlyer in their newest report shows that the global uninstall rates for apps in a month account for about 28 percent. Timely maintenance can come to the rescue by promptly applying all the first time fixes to the app, which retain your active users lowering the chances of uninstalling.
- Sustained User Loyalty – Constant maintenance does finally pay off via sustained user loyalty across the app’s entire lifetime, which is one crucial ingredient that defines your app’s success in the long run.
- High Rankings in Store Listings – Keeping up with ongoing maintenance ensures that your app adheres to quality guidelines and is constantly optimized based on updates and user feedback for properly ranking in the store listings.
- Adaptation Centered on User Feedback – Recurrent maintenance helps monitor usage patterns to retrieve business insights and create individual user funnels. Using in-app analytics tools, developers can analyze the app’s functioning and resolve any crashes or bugs for flawless user experience and improved retention.
- Increased ROI – With a solid maintenance plan in place, your app can achieve long term benefits for your business by reaping higher financial gains, leading to increased ROI. Besides, you can decrease the overall costs by monitoring the app post-launch and removing redundant features that most users ignore or skip by.
Conceive a maintenance plan by collaborating with your app development partner
Finding a reliable app developer with expertise in building enterprise applications helps kick start your app’s development and maintenance cycle. Once signed up, the development team assesses your app’s performance after launch. Taking incoming feedback from users and identifying the potential issues mentioned, the team adopts an end-user development approach to detect bugs and other performance issues beforehand and proactively fix them.
You can get the best in class maintenance support for your mobile app with our application development services. Talk to our consultants today and see how Fingent helps you conceive the right maintenance strategy for your app that yields new revenue streams and enhance your business outcomes.
Stay up to date on what's new
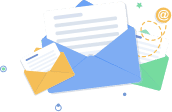
Featured Blogs
Stay up to date on
what's new
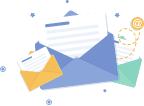
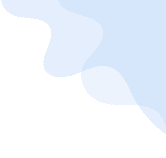
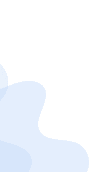
Talk To Our Experts
4 Top Reasons for Organizations to Move to Python 3
Python is one of the most widely used programming languages on the planet. Over the years programmers have fallen in love with Python for its increased productivity and capabilities. However, a constant argument persists on whether Python 3 is better than Python 2 and would it be a wiser decision to completely shift to Python 3.
Although Python 3 has been in existence for over 7 years, programmers are quite skeptical about using it. Most programmers tend to cling to Python 2, completely ignoring the capabilities of the new version.
This blog will walk you through some major drawbacks of Python 2 and will pinpoint 4 top reasons on why to choose Python 3 over Python 2.
Why Is Python 3 On The Rise?
Before we dive deeper into the major advantages of Python 3, let’s take a look at its origin.
Released in 2008, Python 3 was introduced to overcome the flaws of Python 2. The major reason behind developing Python 3 was to clean up the codebase and remove redundancy. Although Python 3 is the newer version of Python, it is not necessarily backward compatible with code written in the 2.x version.
Significant features of Python 3 makes it simpler, easier and incredibly efficient to use. Here’s listing the major differences between Python version 3.0 and 2.0, and the reasons why Python 3 can make a better programming partner.
1. Unicode Character Encoding
As mentioned earlier, Python 3 was introduced to address the vulnerabilities and drawbacks of Python 2. Hence, it reduces the complexities of coding and improves speed and performance.
Where in Python 2 the character encoding is done in ASCII format, Python 3 is Unicode based. In Python 2 the strings are by default stored in the form of ASCII values. Programmers are required to add ‘u’, to store strings specifically in Unicode format. In Python 3 strings are stored in UTF-8 format, enabling a large number of storage features such as character value storage, different language characters, and emojis storage as well.
Dropped deprecated features which were frequent sources of bugs in Python 2 have also been replaced by superior alternatives and retained solely for backward compatibility.
For instance: If a file is created by олга with non-ASCII characters in the name, for the below-mentioned code, if you are using Python 2 your code is sure to throw an error 500.
However, in Python 3 your error will be detected right away saving you the time on long code creation. Moreover, the error message is much easier to understand. Knowing that str object is owner and node is a bytes object, it is easy to recognize that the error is due to listdir returning a list of bytes objects.
Adding listdir(‘.’) would make the bug disappear as this would appear as a Unicode string in Python 3.
The difference in the behavior is due to the difference in how each version handles the string type. Whatever is lumped together in Python 2 is split in Python 3.
2. Improved Library Standards
When it comes to libraries, there is a huge difference between the two versions. Many libraries developed in Python 2 are not forward compatible. Hence, the new 3.x version is developed focusing on providing good compatibility. Moreover, most of the actively maintained libraries are strictly created for use with Python 3. Hence, it’s suggested to keep codes compatible with Python 3 to help keep your test running on both versions.
3. Improved Integer Division
Reducing a programmer’s confusion and frustration, Python 3 is created with a syntax that’s more intuitive. Python 3.x version has an elegantly designed structure that allows performing an action with fewer lines of code. Python 2, on the other hand, requires the exact input to perform a particular result or generate the expected results.
For instance: If you try a simple calculation like 5/2 (5 divided by 2), Python 2, after rounding up would give you the result as 2. To derive the exact result, that is 2.5, the input should be 5.0/2.0.
Whereas, Python 3 would right away, give you the answer 2.5 for the input 5/2, without converting the numbers to float data type.
4. End of Python 2 Support
Yes! Python 2 is expected to stop all support and maintenance by January 2020. Now, this is another major reason why you need to shift to Python 3 at the earliest. By end of support, we mean that all-new packages will be built on Python 3 and hence, it will be difficult to add any new features to the existing Python 2 projects. Major plugins are also being ported to Python 3 and thus, the upcoming updates of these plugins will be available only for the 3.x version.
Moving forward, it will be difficult to find any Python 2 support services or developers. Also, the Python 2 hosting options will grow more scarce and costly. The Python 3.x version and the releases ahead are believed to have different syntax from that of the current version and thus, getting upgrades for existing features would be difficult to find.
Why Should You Stop Using Python 2?
Although programmers have widely accepted and loved working with the 2.x version of Python, there is quite a huge list of flaws and drawbacks experienced with it. Here’s listing a few of them.
1. Firstly, as mentioned in this post, the Python 2 text model is not Unicode capable. It doesn’t handle non-ASCII files correctly. This is one of the major drawbacks of the version. Python 2 handles Unicode module names quite inconsistently, which is a source cause of multiple programming errors. That is why 3.x version of Python is designed to have a Unicode based string type by default.
2. In addition to not being Unicode capable, there is a large number of Unicode handling bugs in Python 2 standard library that might never be fixed. Fixing these bugs within the constraints of Python 2 is too difficult, and not worth the effort.
3. Python 2 iterator was designed long before the introduction of the iterator protocol. Thus, it has a lot of unnecessary and lengthy listings, which can now be made more memory efficient.
4. Programmers who have been involved with Python 2 for a long time might have noticed that the version interprets numbers in a strange way if they have leading zeros. Also, the version has two different kinds of integers. Python 2 beginners are often surprised to find that the version can’t do basic arithmetic correctly.
5. The print and exec statement is also weirdly different from the normal function calls like eval and execfile. Moreover, you need parentheses to catch multiple exceptions.
6. Although list comprehensions are one of Python’s most popular features, surprising errors arise on the local namespace. Also, if you tend to make a mistake in handling the errors, there might be chances where you’ll lose the original error.
Eliminating all these persisting errors and flaws of Python 2, the new version 3.x is specifically designed to enhance the quality and efficiency of the programmers. Thus, it is highly recommended to start preparing for a complete shift to Python 3. For developers who would like to check on to the Python 3 upgrade packages, here’s the command you can use:
[Don’t forget to create a test-requirement.txt file when using the command.]
With increased competition and high consumer expectations, programmers are under constant pressure to improve software performance. With the efficiency and ease of use offered by Python 3, programming is sure to achieve greater success than before. Although Python 2.7 will be supported until 2020, the sooner the switch, the better.
If you are looking for a technology partner to help your business transform with the latest digital trends, then get in touch with our experts today!
Stay up to date on what's new
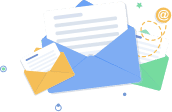
Featured Blogs
Stay up to date on
what's new
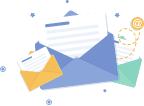
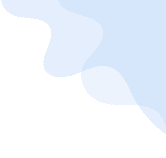
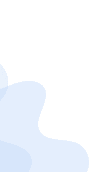
Talk To Our Experts
Data Mining Vs Predictive Analytics: Learn The Difference & Benefits
With big data becoming the lifeblood of organizations and businesses, data mining and predictive analytics have gained wider recognition. Both are different ways of extracting useful information from the massive stores of data collected every day. Often thought to be synonyms, data mining and predictive analytics are two distinct analytics methodologies with their own unique benefits.
This blog examines the differences between data mining and predictive analytics.
Difference Between Data Mining and Predictive Analytics
Data mining and predictive analytics differ from each other in several aspects, as mentioned below:
Definition
Data mining is a technical process by which consistent patterns are identified, explored, sorted, and organized. It can be compared to organizing or arranging a large store in such a way that a sales executive can easily find a product in no time. Various reports state that by 2020 the world is poised to witness a data explosion. Therefore, data mining is a strategic practice that is necessary for successful businesses. It helps marketers create new opportunities with the potential for rich dividends for their businesses.
Predictive analytics is the process by which information is extracted from existing data sets for determining patterns and predicting the forthcoming trends or outcomes. It uses data, statistical algorithms, and machine learning techniques to identify the likelihood of future outcomes based on historical data. In other words, the aim of predictive analytics is to forecast what will happen based on what has happened.
Techniques and Tools
Although there are many techniques in vogue, data mining uses four major techniques to mine data. They are regression, association rule discovery, classification, and clustering. These techniques require the use of appropriate tools that have features like data cleansing, clustering, and filtering. Python and R are the two commonly used programming languages in data mining.
Unlike data analytics, which uses statistics, predictive analytics uses business knowledge to predict future business outcomes or market trends. Predictive analytics uses various software technologies such as Artificial Intelligence and Machine Learning to analyze the available data and forecast the outcomes.
Purpose
Data mining is used to provide two primary advantages: to give businesses the predictive power to estimate the unknown or future values and to provide businesses the descriptive power by finding interesting patterns in the data.
Predictive analytics are used to collect and predict future results and trends. Although it will not tell businesses what will happen in the future, it helps them get to know their individual consumers and understand the trends they follow. This, in turn, helps marketers take necessary, action at the right time, which in turn has a bearing on the future.
Related Reading: Predictive Analytics: The Key to Effective Marketing and Personalization
Functionality
Data mining can be broken down into three steps. Exploration, wherein the data is prepared by collecting and cleaning the data. Model Building or Pattern Identification by which the same dataset is applied to different models, thus enabling the businesses to make the best choice. Finally, Deployment is a step where the selected data model is applied to predict results.
Predictive analytics focuses on the online behavior of a customer. It uses various models for training. With the use of sample data, the model could be trained to analyze the latest dataset and gauge its behavior. That knowledge could be further used to predict the behavior of the customer.
Talent
Data mining is generally executed by engineers with a strong mathematical background, statisticians, and machine learning experts.
Predictive analytics is largely used by business analysts and other domain experts who are capable of analyzing and interpreting patterns that are discovered by the machines.
Outcome
Data mining enables marketers to understand the data. As a result, they are able to understand customer segments, purchase patterns, behavior analytics and so on.
Predictive analytics helps a business to determine and predict their customers’ next move. It also helps in predicting customer churn rate and the stock required of a certain product. Additionally, predictive analytics enable marketers to offer hyper-personalized deals by estimating how many new subscriptions they would gain as a result of a certain discount, or what kind of products do their customers seek as a complement to the main product they bought from the seller.
Related Reading: Using Predictive Analytics For Individualization in Retail
Effect of Data Mining and Predictive Analytics on the Future
The global predictive analytics market is estimated to reach 10.95 billion by 2022. We are now in a period of constant growth, where businesses have already started using data mining and predictive analytics sift through the available data for searching patterns, making predictions and implementing decisions that will impact their business.
Both approaches enable marketers to make informed decisions by increasing productivity, reducing costs, saving resources, detecting frauds, and yielding faster results. To make the best use of data mining and predictive analytics, you need the right guidance and the best expertise. Talk to our experts and find out how Fingent can help your business scale up with the power of data. Get on your way to a digital-first future with Fingent.
Stay up to date on what's new
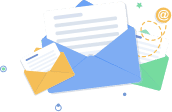
Featured Blogs
Stay up to date on
what's new
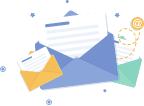
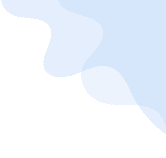
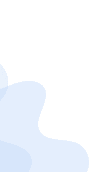
Talk To Our Experts
Can Data Warehousing Enhance the Value of Data Visualization & Reporting?
Organizations rely heavily on data to make crucial business decisions. Hence, it is important for your business to have access to relevant data. That is where a well-designed data warehousing comes to your rescue!
Besides gaining actionable insights, corporate executives, business managers, and other end-users make more informed business decisions based on historical data.
Today’s Analytics and Business Intelligence solutions provide the ability to:
- Optimize business processes within your organization
- Increase your operational efficiency
- Identify market trends
- Drive new revenues
- Forecast future probabilities and trends
Before understanding how data warehousing can add more value to data visualization and reporting, let’s take a look at what these terms mean.
Analytics and Business Intelligence
Business Intelligence is a process that includes the tools and technologies to convert data from operational systems into a meaningful and useful format. This helps organizations analyze and develop meaningful insights to take timely business decisions. The information derived from these tools demonstrate the root cause of your business problems and allow decision-makers to strategize their plans based on the analysis.
Business Intelligence is information not just derived from a single place, but multiple locations and sources. It can be a combination of the external data derived from the market and the financial and operational data of an organization that is meaningfully applied to create the “intelligence”.
Data Warehouse
Data warehouse is a repository that collects data from various data sources of an organization and arranges it into a structured format. An ideal data warehouse set up will extract, organize, and aggregate data for efficient comparison and analysis. Data warehouse supports organizations in reporting and data analysis by analyzing their current and historical data. This makes it a core component of Business Intelligence.
Unlike a database, that stores data within, at a fully normalized or third normal form (3NF), a data warehouse keeps the data in a denormalized form. It means that data is converted to 2NF from 3NF and hence, is called Big Data.
Key benefits of a Data Warehouse
- Combine data from heterogeneous systems
- Optimized for decision support applications
- Storage of historical and current data
Why We Need Data Warehouse for Business Intelligence?
Before the business intelligence approach came into use, companies used to analyze their business operations using decision support applications connected to their Online Transaction Systems (OLTP). Queries or reports were retrieved directly from these systems.
However, this approach was not ideal due to:
- Quality issues
- Reports and queries were affecting business transaction performance
- Data resides in heterogeneous sources
- Non-availability of historical data
- Non-availability of data in the exact form required for reporting
Connecting your organization’s business intelligence tools to a data warehouse can provide you benefits in terms of production, transportation, and sale of products.
Data Visualization vs. Data Analytics – What’s the Difference?
Data Warehousing and Business Intelligence Using AWS
Today, traditional BI has given way to agile BI where agile software development accelerates business intelligence for faster results and more adaptability. Big Data is growing fast to provide useful insights for making improved business decisions.
There has been a paradigm shift in data storage with warehousing solutions moving increasingly to the cloud. Amazon Redshift, for instance, is one of the most popular cloud services from Amazon Web Services (AWS). Redshift is a fully-managed analytical data warehouse on cloud, that can handle petabyte-scale data, which enables analysts to process queries in seconds.
Redshift offers several advantages over traditional data warehouses. It provides high scalability using Amazon’s cloud infrastructure to set-up and for maintenance, without the need for upfront payments. You can either add nodes to a Redshift cluster or create additional Redshift clusters to support your scalability needs.
You can use AWS Marketplace ISV Solutions for Data Visualization, Reporting, and Analysis.
Data visualization helps you identify areas that need attention or improvement, clarify factors that influence business such as customer behavior, and making decisions such as finding out a suitable market for your product or predicting your sales volumes, and much more.
TIBCO Jaspersoft, for example, is a solution that delivers embedded BI, production reporting, and self-service reporting for your Amazon data at affordable rates. It features the ability to auto-detect and quickly connect to Amazon RDS and Amazon Redshift. Jaspersoft is available in the AWS Marketplace in both single-tenant and multi-tenant versions. TIBCO Jaspersoft for AWS includes the ability to launch in a high availability cluster (HA) as well as with Amazon RDS as a fault-tolerant repository. Pricing is based on the Amazon EC2 instance, type as well as the chosen single or multi-tenant mode.
Image source: http://bit.ly/2IWWCDn
Summary
By moving your analytics and business intelligence to a hybrid cloud architecture you will be able to handle huge amounts of data and scale at the rate of expansion required by your business. You will also be able to deliver information and solutions at the speed that your employees and customers demand, and gain insights that will enable your organization to innovate faster than ever.
Business Intelligence and Data Warehousing are two important aspects of the survival of any business. These technologies give accurate, comprehensive, integrated, and up-to-date information on the current enterprise scenario which allows you to take the required steps and make crucial decisions for your company’s growth. To know how your business can benefit from the latest technologies, get in touch with our experts today
Stay up to date on what's new
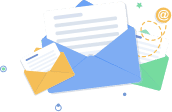
Featured Blogs
Stay up to date on
what's new
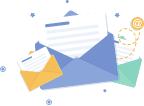
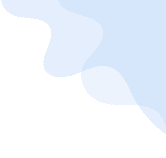
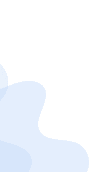
Talk To Our Experts
How Chat Bots Can Enhance Student Onboarding
Conversational interfaces have gone mainstream. The technology behind keeps crossing new milestones, the result of which chatbots have transformed from simple Q&A systems to intelligent personal assistants. As a result, bots found widespread application in diverse areas, most recently in education.
Although education stayed backward in terms of technology adoption, lately it took on a renewed quest to incorporate it. Educators are on the lookout for innovative ed-tech systems for efficient tutoring and students increasingly prefer personalized learning environments.
Deploying chatbots at numerous front-ends like college/university websites, internal student communication portals or even popular instant messaging platforms can help with that. Here’s how?
Chatbots bring in a personalized and engaging learning experience optimized to the learning pace of each learner, which actively drives student-centered learning at the forefront. Configuring a bot to answer student inquiries related to curriculum, courses, admissions, etc. as well as deliver learning resources on request makes way for a personal always available assistant that every student can engage with.
That’s exactly what we did, though in a different way.
Recently Fingent was approached by a client, a leading public research university based in Australia to develop an intelligent chatbot for assisting prospective and freshly enrolled candidates with onboarding and orientation, course-related information, credit scores, etc. The client wanted to streamline its entire student orientation process using a chatbot and make all related information better accessible and context-based as well as systematically tackle the ‘summer melt’ rates.
Here, we lay down a high-level abstract of this chatbot development experience powered by IBM Watson Assistant and backed by .NET Core. It briefs various facets and challenges faced during the design and development of the system.
The Plot (Objective)
“Build a chatbot to assist candidates during the orientation process of Monash University. The chatbot should be capable of handling different context-based scenarios such as listing available courses, providing credit score information, course structure, projects associated with each course and many more.“
Since it is a Proof of Concept (POC) project, and Monash University offers a wide range of courses based on various areas of education, the team decided to choose one particular area and focus on only two of the selected courses (Bachelor of Accounting & Bachelor of Actuarial Science). This is to repress the scope in control, considering the timespan and resource availability.
Foundation
Keeping in mind the idea of building a highly sophisticated chatbot, an ideal and matured chatbot assistant technology had to be finalized, which provides both comprehensive user intent identification and processing as well as a satisfactory response according to the user query. The system should also provide an extensive and less technicality included training interface. The hunt for such a tech ended up in IBM Watson Assistant.
Terminologies
The world of chatbots has some common terms which are essential key knowledge required while developing a chatbot. We can call them as the pillars of a chatbot.
- #Intent – Intent is nothing but the user’s intention in a query – basically covers all types of questions and their varieties, the user probably may ask. This can be queries within the scope or related to the scope.
Examples:
“What are all the courses available?”
– Intent associated: #KnowCourseInfo
“How much credit I require in the first semester?”
– Intent associated: #KnowCreditInfo
Remarks – There will be some stock #intent collection depending upon the chatbot engine, which is designed to handle the general greetings and conversation-oriented chunks. We can import or enable the intents as we want to make our chatbot more conversational and human-friendly.
- @Entity – An entity is a subject addressed in the user query. There are mainly two categories of entities. They are Scope-based entities and System entities. Scope-based entities are entities that belong to the scope we address whereas System entities are “primitive system-aware” entities.
Examples:
“What are all the courses available?”
– Entities associated: @Course
“How much credit I require in the 1st semester?”
– Entities associated: @Credit, @Semester, @system_number:1st
Remarks – On diving deeper, we may need the support of multiple types of scope-based entities and a system-aware way of specifying the relationship between the entities (which lacks in IBM Watson Assistant). This is to specify the entity characteristics as more descriptive as well as with the notion of “the system knows” the given attributes and relationships of an entity.
- Dialog – A dialog is a declarative way of specifying the possible questions the user may ask, and how should the bot respond to the corresponding questions. Generally, this will be a tree-based structure, rooted in the key user intentions and scope covered features. We will be handling the different scenarios of a single #intent as well as the edge cases.
- $Context Variable – A context variable is to store information, collecting from a dialog context or it can be any information related to the dialog context. It helps us to keep the dialogue context and facilitates conversational flow.
- Skill/Workspace (IBM Watson based) – A skill is a package that consists of the above-mentioned factors, in which all are aligned into a single chatbot capability, in our case, it was Onboarding skill.
Implementation
The entire development process streamlined into two major sections. The first one is aligned to the chatbot engine intelligence building and improvement activities while the other one is for the middleware and UI development.
1. Intelligence Build-up on top of IBM Watson Assistant
- Analyzed the requirements and fixed the boundaries of the scope. It includes what all are the functional areas to be covered by the proposed chatbots.
- Prepared the possible user queries and categorized them as #intents.
- Identified the underlying @entities in each question and classified them to form the actual set of primitive entities.
- Designed the dialog structure based on the prepared user query sets. See the resources: Intent structure and Dialogue flow.
Fig 1. Intent Structure
- Continuously refined the dialogue structure based on detecting each edge cases and to incorporate new scenarios.
- Used some conventions on responses to extend the chatbot response capabilities, according to the requirements. This is to handle specific use cases such as clickable action list image response, map response, and show a list of items.
- Implemented WebHooks (IBM Watson based) to talk to external APIs to fetch the values for a dialogue node as well as validating user input (Not a comprehensive solution).
2. Middleware and UI Development
- Built a middleware backed by .NET Core with an intention to plug any chatbot service to the UI module. In fact, it is designed as a standard-framework to separate the chatbot logic from the application logic. This enables hassle-free maintenance of the app logic, code reusability, and extensibility.
- Built the UI using Angular to provide a sophisticated face for our chatbots.
Fig 2. Dayton Interface
Also, we built a diagnostics module, as part of the UI, which provides the service configuration information and session-based transcripts of conversations held with the chatbot.
Fig 3. The architecture of the Chatbot Middleware Application, Source Code
Challenges
During the development, we came across some development challenges with IBM Watson, which are listed below.
- Unable to map relationships between entities. Due to this limitation, we were unable to link and pull the related values of the entities.
- Conflicts between various entity values (Solved partially via entity split-up method)
- API Limitations to manage chatbots dialog schema
- IBM Watson doesn’t provide active learning, the self-learning capability to learn from user conversation sessions.
- It also doesn’t provide an efficient way to talk to external APIs. Only one external API can be called, which leads to a bottle-neck on executing the webhook actions.
- No built-in user input validation. This has to be done via WebHooks.
Capitalizing on AI Chatbots Will Redefine Your Business: Here’s How
Final Words
The application is now in a showcase/UAT (User Acceptance Testing) mode, also the refinement process being in progress. It has miles to go to reach the capability to converse with the user as a comprehensive onboarding assistant.
To know how chatbots can enhance your business growth, get in touch with our experts today!
Stay up to date on what's new
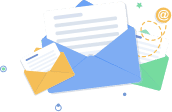
Featured Blogs
Stay up to date on
what's new
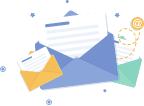
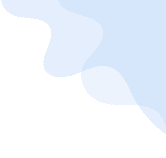
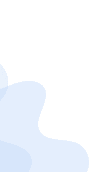
Talk To Our Experts
Mixed Reality Promising a New Improved Healthcare Industry
Mixed Reality makes it possible for surgeons to perform an operation on a patient thousands of miles away. It makes it possible for nurses to hone their skills and perform hundreds of operations virtually before even touching a patient. Mixed Reality is making the impossible possible.
Mixed Reality combines the real world with the virtual to create solutions that we never thought possible. It enables effective collaboration between physical and digital objects and has found an integral place and application in healthcare. This blog explores some radical ways in which healthcare is using Mixed Reality for better quality treatment and customer experience.
Mixed Reality Working Wonders in Healthcare
By 2026, AV/VR in the healthcare market is expected to reach 7.05 billion USD showing that Mixed Reality is finding increased application in the healthcare industry. From enabling pre-procedural planning and visualization before surgery to training nurses as they virtually workout challenges they could encounter during real-life procedures, and improving the collaboration and communication between doctors and patients – Mixed Reality is making great things happen. Here are some ways in which this is becoming possible.
1. Immersive Learning for Nurses and Medical Students
Skilled nurses are critical to the healthcare industry. Simulations are the most effective method of educating and preparing nurses to respond appropriately to a variety of situations they might encounter. Mixed Reality can place a nursing student in those specific or rare situations, which may be difficult to arrange for in real clinical settings. Such immersive simulations are much more cost-effective than traditional nursing simulation devices. The education company Pearson has collaborated with Microsoft to launch apps called HoloPatient and HoloHuman. These tools use holograms of patients and help in training healthcare professionals as they diagnose and treat medical problems.
As another achievement in Mixed Reality, St. George’s University in Grenada worked with SphereGen Technologies to develop what is called the ‘Learning Heart.’ The Learning Heart is a study aid that enables users to view the hologram of the heart from all directions and examine its functions. It responds to touch and voice commands and allows users to separate all the parts of the human heart, thus making learning immersive for medical students.
2. Reduced Time and Reduced Human Error in Reconstructive Surgery
Reconstructive surgeries enabled by Mixed Reality with the use of HoloLens have proven to be very successful at the Imperial College at St. Mary’s Hospital, London. According to the team guided by Dr. Philip Pratt, Mixed Reality helps surgeons locate and reconnect major blood vessels. With HoloLens, surgeons are able to use holographic overlays to see the bones and identify the course of blood vessels which aids them in their surgery, improving the outcome for the patient. An article in The Times entitled Holograms to get surgeons under the skin of patients showed examples of a 41-year-old man and an 85-year-old woman on whom such reconstructive surgeries were performed successfully.
3. Revolutionizing Surgery
In December 2017, Dr. Thomas Gregory undertook a live transplant surgery with the help of HoloLens. It helped him access the patient’s medical information and anatomical pictures in 3D during the surgery. Since HoloLens is a standalone computer worn like a helmet by the surgeon, his hands are free for surgery. Additionally, the use of microphones and sensors allows the surgeon to communicate with other surgeons in different parts of the world making collaboration easier. All these features, along with the simulations and information it can pull up, make Mixed Reality a valuable asset in improving surgical performance.
4. Improving the Patient Experience
Building trust through efficient communication is an important aspect of a doctor-patient relationship. Mixed Reality makes this possible in a more immersive way. For example, in a recent interview with Sirko Pelzl, CEO and CTO of apoQlar, he spoke about Virtual Surgery Intelligence (VSI) and said: “physicians can use VSI to show patients their own MRI scans and explain the surgical procedure in visual detail. We were able to illustrate in a recent study how greatly patients appreciated this education and communication.”
Mixed Reality also helps in reducing response time and improving surgical accuracy, which contributes to the smooth and successful patient experience. For example, when the surgery is complicated or the patient is critical, diagnostic images with Mixed Reality can serve as a twin of the patient. This helps doctors discuss, plan, and walk through their treatment protocol, thus reducing response time in patient care.
Mixed Reality also gives doctors all the information they need about a patient and enables real-life simulations to help him in his decisions and actions. Commenting on a prototype application called “Cinematic Rendering for Surgery” Christian Zapf, head of the Syngo Business Line at Siemens Healthineers said, “The error rate dropped from 14.1 to 0.8 percent for surgeons in training and from 11.1 percent to 0.8 percent for qualified surgeons.”
https://www.fingent.com/insights/portfolio/future-communication-security-using-augmented-reality/
The Future of Mixed Reality
Mixed Reality has already made deep inroads, especially in medicine. It will continue to improve the quality of healthcare and medical training as it positively impacts the medical industry. Fingent has helped many clients build custom healthcare software solutions, which integrate applications with the latest technologies like Mixed Reality. Get in touch with us and let’s discuss how we can make Mixed Reality possible for you.
Stay up to date on what's new
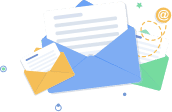
Featured Blogs
Stay up to date on
what's new
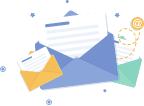
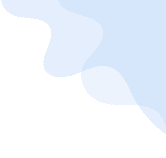
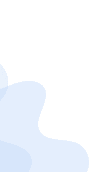